Joseph Spruce, Risa Wu, Russell Berry
GIS Techniques for Evaluating Wetland Maps Derived from Remotely Sensed Data*
ABSTRACT
Wetland delineation remains a problematic issue for governmental agencies involved with environmental monitoring. Remotely sensed data, particularly aerial photographs, are important for mapping wetlands at regional scales. New digital remote sensing technologies (e.g., medium- to high-resolution multiband satellite and airborne imagery of visible, near-infrared, mid-infrared, thermal, and radar spectral regions) may improve National Wetlands Inventory (NWI) mapping efforts by increasing map accuracy and reducing map production costs. In response, NASA's Commercial Remote Sensing Program Office performed a study to evaluate the utility of commercially available remote sensing technology for deriving wetland classification and mapping
products for the NWI. This paper discusses GIS techniques used to assist this investigation. This project involved processing each remotely sensed data set into wetness maps for comparisons
against NWI maps and GPS-based field survey data. GIS tools found in ArcInfo software proved to be indispensable for quantifying and visualizing comparisons of test and reference wetness maps. GISs also assisted in wetness signature evaluation and development by enabling analysts to cross-tabulate unsupervised classifications of remotely sensed data with NWI wetness maps.
INTRODUCTION
This paper discusses the use of advanced GIS software, such as ArcInfo and ERDAS Imagine,
for aiding the analysis of wetland maps derived from remotely sensed data. In particular, this
paper shows how geographers can use remotely sensed data and Geographic Information
Systems to compare and analyze spatial wetland data.
Wetland delineation remains a problematic issue for governmental agencies involved with
environmental monitoring. Remotely sensed data, particularly aerial photographs, are important
for mapping wetlands at regional scales. New digital remote sensing technologies (e.g., medium-
to high-resolution multiband satellite and airborne imagery of visible, near-infrared, mid-
infrared, thermal, and radar spectral regions) may improve National Wetlands Inventory (NWI)
mapping efforts by increasing map accuracy and reducing map production costs. NASA's
Commercial Remote Sensing Program Office performed a study to evaluate the utility of
commercially available remote sensing technology for deriving wetland classification and
mapping products for the NWI. This paper discusses GIS techniques used in this study.
Remotely sensed data products often constitute an invaluable component of GISs, and a great
deal of software development has taken place to aid the use of remotely sensed data in GISs.
Modern GISs give users the ability to conduct visual and quantitative analysis involving multiple
kinds of digital spatial data, including remotely sensed imagery. In addition, GISs provide
several tools for working with raster remotely sensed and thematic imagery. For example, a
screen display of remotely sensed imagery with co-registered spatial data can enhance spatial
data visualization and interpretation. GISs also allow quantitative comparisons between
classified maps from remotely sensed data and other thematic data sets. In addition, some of the
more advanced GIS software packages (e.g., ArcInfo) now include basic functions for
processing and analyzing remote sensing data, such as image screen displays, georeferencing,
and classification.
OBJECTIVES AND DATA ACQUISITION
The primary objective of the study was to evaluate the utility of high-resolution multispectral optical and radar imagery for discriminating wetland from upland sites. The investigators selected Salisbury, Maryland, as the focal area for this study because it contains numerous transitional forested wetlands that are difficult to map from aerial photography. The project investigators evaluated several remotely sensed data sets, including: 1) Airborne Terrestrial
Applications Sensor (ATLAS) airborne daytime 2.5-meter multispectral imagery with bands in the visible, near-infrared, and thermal-infrared spectral regions; 2) ATLAS nighttime 2.5-meter multiband thermal imagery; and 3) AIRSAR airborne 9-meter multiband radar imagery of C, L, and P bands. The research team chose to acquire these data sets because they have high spatial resolutions and spectral information with high potential for wetland identification.
Additional data sets were acquired for evaluation of wetland mapping results: 1) a GPS-based survey of wetland boundaries, 2) GPS-based locations of wells located across field-delineated wetness gradients, 3) National Wetland Inventory digital maps produced at 1:24,000 scale by the U.S. Fish and Wildlife Service (USFWS), 4) 1:24,000 U.S. Geological Survey topographic maps, and (5) 1:8,000 color infrared (CIR) aerial photography acquired in 1995 during the same mission as the airborne daytime multispectral imagery.
METHODS
Table 1 lists several GIS-based data processing routines used to produce and/or evaluate the wetness maps for the study. Remote sensing specialists processed each remotely sensed data set into wetness maps by subjecting each raw data set to unsupervised ISODATA classification into 25 spectral clusters. They also prepared an NWI map from 1989 aerial photography by downloading it from the USFWS Internet site, importing it into ArcInfo, and recoding the soil moisture regime into a wetness map. Each classification from remotely sensed data was cross-tabulated with the NWI wetness classification. Analysts recoded spectral cluster classes as wetland or upland depending on what the majority of the cluster class pixels were on the NWI wetness map. To promote classification success, analysts reclassified imaged areas with less than 70% majority value on the NWI map. Then they applied GIS overlay techniques to merge
initial and secondary classifications into a final classification. Each final classification was then
cross-tabulated against the NWI wetness map to quantify wetland and upland map agreement,
plus map disagreement through wetland omission and commission. In addition, analysts
employed visual and quantitative analysis techniques to compare assorted wetness maps with
field survey data (wetland boundary delineations and well sites).
RESULTS AND DISCUSSION
This project turned out several mapping products. Figure 1 shows the results of raster-based
additive overlays between the NWI wetness classification and the final wetness map from each
remotely sensed data set. Such comparative analyses give an indication of wetland map
accuracy, though NWI maps also have classification errors. The AIRSAR wetness map
(Figure 2) had 71% overall agreement with the NWI map, the best of any individual remote
sensing data set.
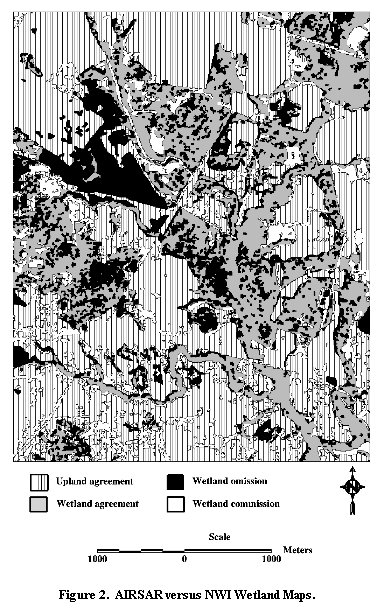
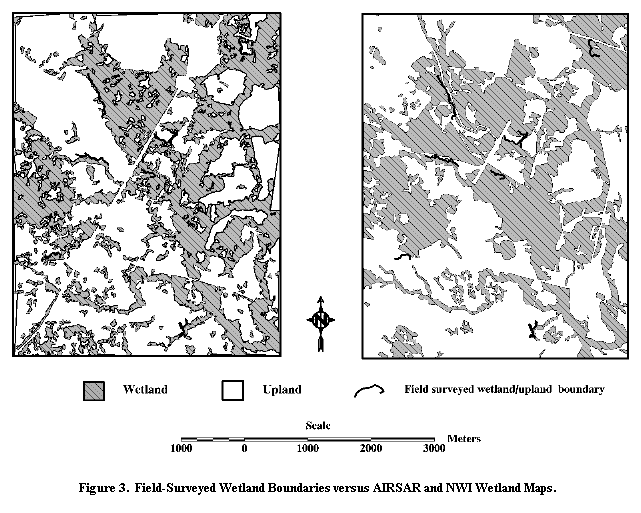
Figure 3 compares the NWI and AIRSAR wetness maps to GPS-based wetland boundary data.
This map composition contains visual evidence that the NWI wetness map has commission and
omission errors. The investigators also cross-tabulated the reference wetland boundary data
against buffered wetness maps from NWI and AIRSAR data (Table 2). Analysis of this table
reveals some similarities and differences between the NWI and AIRSAR wetness maps. In
particular, 95% of the field-surveyed wetland boundaries are within 50 meters of wetlands
mapped by NWI and AIRSAR data. However, only the NWI map has field-surveyed boundaries
(120 meters in total) greater than 100 meters from mapped wetlands. This result lends evidence
to the belief that the NWI contains some omission mapping errors. Such cross-tabulations do not
provide information on commission errors; compiling such information will require additional
GIS processing.
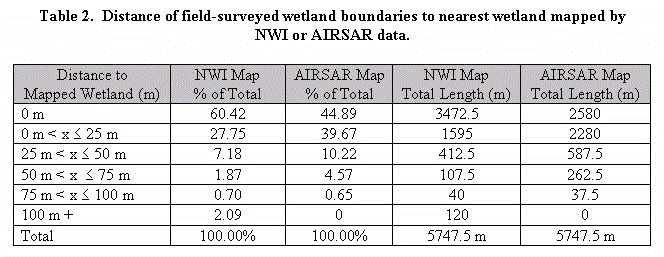
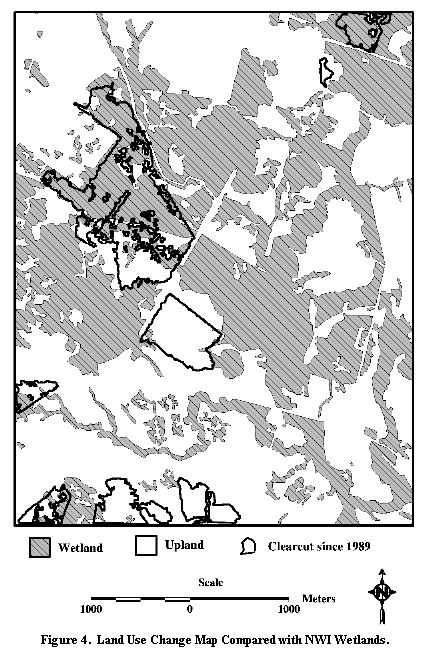
Not all the map disagreement between the NWI and a given test data set is due to classification
error. The NWI map incorporated spring 1989 CIR aerial photography, while this investigation
employed remotely sensed data sets from either spring or early summer 1995. Figure 4 shows a
land-use change map derived from 1989 and 1995 airborne CIR color composite imagery. This
map indicates clearcuts occurred over 10% of the study area from 1989 through 1995. Such land
use change may alter the agreement between wetness maps from remotely sensed and NWI
classifications. In particular, clear-cuts may have changed the soil moisture regime in some of
the areas originally classified as wet on the NWI. Some of the map disagreement may be due to
georeferencing error. In addition, the soil saturation level during data acquisition represents
another influence on wetness map disagreement, since the AIRSAR data was acquired in early
summer when the soils were less saturated than during the spring runoff. Investigating such a
seasonal effect would entail comparison of wetness maps derived from both early summer and
springtime AIRSAR imagery.
Figure 5 compares well sites rated for wetness to wetness maps from NWI and AIRSAR data.
This map composition shows the inadequacy of NWI and AIRSAR wetness maps for site-level
wetland mapping. Neither the NWI or AIRSAR data correlate consistently with the well data,
especially when one considers the transitional wetland and upland wells. However, a much
stronger correlation occurs by considering only non-transitional wetlands and uplands. This
GIS-based interpretive analysis can also be quantified for each wetness map by applying
buffering routines on the wetness map, rasterizing each buffered output, and then cross-
tabulating the gridded buffer file against the well wetness data. Doing so would provide an
estimate of the proximity of each well to the wetland boundary. This procedure was not
performed due to time constraints and the small number of wells.
Overall, this project yielded some encouraging results indicating certain kinds of remotely sensed data (particularly AIRSAR imagery) have potential for mapping wetland versus upland areas.
However, these preliminary findings require validation in the form of an intensive, statistically
rigorous map accuracy assessment. The NWI data worked well as a data set for guiding the
production of wetland maps from remotely sensed data but have limitations as an ultimate
reference data set. The available in situ data on wetland boundaries were useful but limited by
the comparatively small sample size and non-random selection of sampling sites. An intensive
accuracy assessment involves laborious field surveys performed by soil scientists and equates to
a significant outlay of cash. Such work is needed to learn more about the nature of agreement
between wetness maps from the NWI and those from remotely sensed data. Perhaps a good
strategy would be to field survey a stratified random sample of wetland versus upland points
from the NWI map. Many GIS software packages have the tools to perform this kind of
operation.
CONCLUSIONS
The wetness map from AIRSAR imagery has greater overall agreement (71%) with the NWI
wetness classification compared to ATLAS daytime multispectral data (63%) and ATLAS
nighttime thermal data (59%). GIS-based comparisons of test wetness maps to NWI maps
enables analysts to visualize and quantify agreement and disagreement in map areas. However,
such comparisons should be cautiously viewed as aids for map accuracy assessment because
1) NWI maps have classification errors, 2) significant land use change has occurred in the study
area since construction of the NWI maps in 1989 (10% of the forests were clearcut), 3) each
wetness map has a different amount of georeferencing error (i.e., positional accuracy), and 4) the
AIRSAR data set is not from the same season as the other data sets.
Comparisons of wetness maps with the in situ wetland data indicate both the AIRSAR and NWI
wetness maps do not map wetlands to the same level of precision as GPS-based surveys by soil
scientists. The results from this study suggest that the AIRSAR and NWI data tend to map
wetlands within 50 meters of the field-surveyed wetland boundaries. This level of wetland
mapping precision (for the AIRSAR and NWI maps) does not suffice for site-level delineation
but can be adequate for regional surveys. GIS analysis also shows that some NWI wetlands are
greater than 100 meters from field-measured wetland boundaries. Such anomalies probably
indicate wetland omission error. However, a statistically rigorous map assessment is needed to
estimate the accuracy of wetland maps from NWI and AIRSAR data. In addition, the wetland
mapping approaches used in this study should be tried and evaluated on other kinds of
landscapes, such as coniferous forested wetlands in the western United States. Doing so would
further validate these wetland mapping technologies.
GIS tools, such as those found in ArcInfo software, proved to be indispensable for quantifying
and visualizing comparisons of assorted wetland data. In particular, this study incorporated GIS
to generate wetland maps from remotely sensed data and to compare the results to reference NWI
maps and in situ wetland boundary data. GISs also assisted in wetness signature evaluation and
development by enabling analysts to cross-tabulate unsupervised classifications of remotely
sensed data with NWI wetness maps.
Today, the growth in GIS-based functionality has increased the possibilities for using remotely
sensed imagery in GIS-based analyses. The analyses for this wetland study benefited immensely
from the use of the many raster data processing functions now residing in full-capability GIS
systems, such as ArcInfo and ERDAS Imagine. As a result, those working with remotely sensed
data are finding that GIS can actually help mapping specialists build and evaluate thematic data
sets from remotely sensed data. In addition to full-fledged GIS software, most of the major
desktop mapping software packages (e.g., ArcView) now provide functions for incorporating
remotely sensed imagery. Also, the amount and kind of commercially available remotely sensed
data continue to grow and will do so into the next century. Such developments should help those
conducting environmental surveys such as the wetland mapping project described here.
ACKNOWLEDGMENTS
This work was supported by the NASA Office of Space Access and Technology, Commercial
Remote Sensing Program Office at the John C. Stennis Space Center, Mississippi. The following
federal agencies contributed personnel and resources towards completion of this work: 1) U.S.
Environmental Protection Agency, 2) U.S. Geological Survey, 3) U.S. Fish and Wildlife Service,
and 4) Natural Resource Conservation Service. In addition, the Jet Propulsion Laboratory, the
Maryland Department of Natural Resources, and Earthsat Corporation also provided timely
support.
* Any use of trade, product, or firm names is for descriptive purposes only and does not imply endorsement by the U.S. Government.